Artificial Intelligence Index Report 2023: An overview
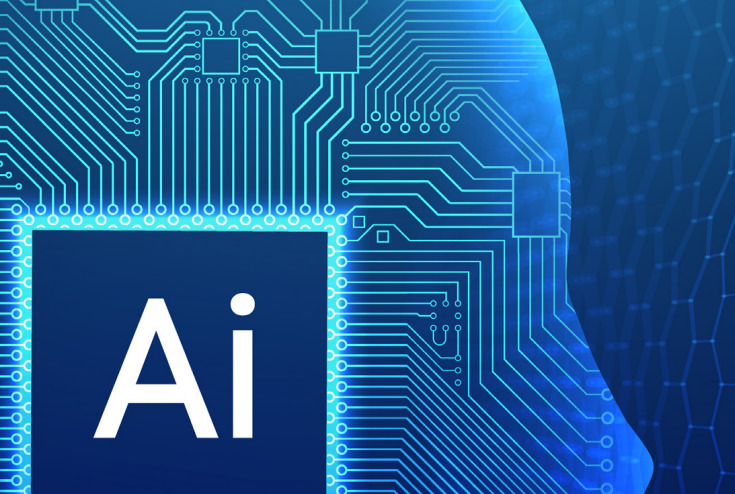
The AI Index Report tracks, collects, distills, and visualizes artificial intelligence data. It aims to give unbiased, rigorously validated, widely sourced data to policymakers, researchers, CEOs, journalists, and the general public to enable them to build a more thorough and nuanced knowledge of the complicated area of AI.
In trends in Significant Machine Learning Systems, the report highlights that the most common class of system among the important AI machine learning systems produced in 2022 was language. Twenty-three major AI language systems were released, almost six times the amount of the next most prevalent system type, multimodal systems. Until 2014, most machine learning technologies were released by academia. Since then, the industry has gained control. In 2022, there were 32 prominent industry-made machine learning systems compared to only three generated by academia. Producing cutting-edge AI systems increasingly necessitates vast volumes of data, processing power, and money, all of which industry actors have in bigger quantities than nonprofits and academia.
In 2022, the United States had 16 machine learning systems with 285 authors that generated the systems, followed by the UK with eight machine learning systems and China with three systems.
In the previous half-decade, the amount of computing used by large AI machine-learning systems has expanded dramatically. The increasing demand for computing in AI has numerous significant ramifications. More compute-intensive models, for example, have bigger environmental implications, and industrial participants have easier access to computational resources than others, such as universities. Language models have gradually demanded the most computing resources of any machine learning system since 2010.
Large language and multimodal models, also known as foundation models, are a new and growing form of AI model that is trained on massive amounts of data and adaptable to a wide range of downstream applications. The report notes that large language and multimodal models such as ChatGPT, DALL-E 2, and MakeA-Video developed by researchers from Canada, Germany, and India, have shown outstanding capabilities and are beginning to be widely applied in the real world. The number of parameters in freshly published large language and multimodal models has grown dramatically over time. GPT-2, the first major language and multimodal model announced in 2019, had just 1.5 billion parameters. PaLM, which Google launched in 2022, has 540 billion, roughly 360 times higher than GPT-2. Over time, the median number of parameters in big linguistic and multimodal models grows exponentially.
Additionally, it explains that large language and multimodal model training computation has also progressively grown. An example of this is the compute used to train Minerva (540B), a large language and multimodal model released by Google in June 2022 that demonstrated impressive abilities on quantitative reasoning problems, and was approximately nine times greater than that used to train OpenAI’s GPT-3, also released in June 2022, and approximately 1839 times greater than that used to train GPT-2 (released February 2019).
Open-Source AI Software
The report describes the GitHub project as a collection of files that may contain the source code, documentation, configuration files, and images for a 1.4 Open-Source AI Software software project. The overall number of AI-related GitHub projects has continuously expanded from 2011, rising from 1,536 in 2011 to 347,934 in 2022. A high fraction of GitHub AI projects was contributed by software developers in India at 24.2%, the European Union and the United Kingdom came in second at 17.3% followed by the United States at 14.0%.
By “starring” a repository, GitHub users can bookmark or save their interests. A GitHub star, similar to a “like” on a social networking platform, symbolizes support for a certain open-source project. Some of the most popular GitHub repositories include libraries such as TensorFlow, OpenCV, Keras, and PyTorch, which are frequently utilized by software developers in the AI coding community.
The report reviews the below most notable technological breakthroughs in AI since 2022;
ü DeepMind Released AlphaCode, an AI system that writes computer programs at a competitive level.
ü DeepMind Trains Reinforcement Learning Agent to Control Nuclear Fusion Plasma in a Tokamak
ü IndicNLG Benchmarks Natural Language Generation for Indic Languages
ü Meta AI Released Make-A-Scene, a text-to-image AI model that enables users to generate images through text.
ü Google Released PaLM Google’s AI, one of the world’s largest language models.
ü OpenAI Released DALL-E 2 DALL-E 2, a text-to-image AI system that can create realistic art and images from textual descriptions.
ü DeepMind Launched Gato Gato, a new reinforcement learning agent capable of doing a wide range of tasks such as robotic manipulation, game playing, image captioning, and natural language generation.
ü Google Released Imagen, a text-to-image diffusion model capable of producing images with a high degree of photorealism.
ü Authors Across 132 Institutions Teamed Up to Launch Beyond the Imitation Game Benchmark (BIG-bench).
ü GitHub Makes Copilot Available as a Subscription-Based Service for Individual AI systems capable of turning natural language prompts into coding suggestions across multiple languages.
ü Meta Announced ‘No Language Left Behind’ (NLLB), a family of models that can translate across 200 distinct languages.
ü Meta Released Make-A-Video, a system that allows users to create videos from short text descriptions.
ü Meta Released CICERO CICERO, the first AI to play in the top 10% of human participants in the game Diplomacy.
ü OpenAI Launched ChatGPT, an impressive, publicly usable chatbot capable of writing university-level essays.
ü The ability of AI systems to generate synthetic images that are sometimes indistinguishable from real ones has resulted in the production of deepfakes, which are images or movies that appear real but are fake. See our article on deep fakes here https://vellum.co.ke/era-of-deepfakes-risks-and-the-need-for-protective-laws-an-explainer/
Concerns about the environmental impact of computational resources and the energy necessary for AI training and inference have grown. Although there is no universal standard for measuring the carbon intensity of AI systems, the report synthesizes the findings of several researchers investigating the relationship between AI and the environment and notes that as AI models get larger and more widely deployed, they will become increasingly vital for the AI research community to consciously assess the impact of AI.
The AI models pose ethical issues. According to the AIAAIC database, which analyzes occurrences involving the ethical misuse of AI, the number of AI incidents and controversies has increased 26 times since 2012. In 2022, major happenings included a deep fake video of Ukrainian President Volodymyr Zelenskyy surrendering and US jails utilizing call monitoring technology on their detainees.
Additionally, Intel is collaborating with the education firm Classroom Technologies to develop an AI-based solution that can detect students’ emotional states on Zoom. There are privacy and discrimination problems associated with the usage of this technology, there is a danger that pupils will be overly observed and that systems may mischaracterize their feelings.
Further, the London Metropolitan Police Service supposedly keeps a database of over a thousand street gang members dubbed the Gangs Violence Matrix (GVM) and utilizes AI technologies to rank the danger potential of each gang member. Several kinds of research have indicated that the GVM is inaccurate and discriminates against specific ethnic and racial minorities. Several ethical concerns have also been raised about Midjourney, including copyright (the system is trained on a corpus of human-generated images without attribution), employment (concerns that systems like Midjourney will replace the jobs of human artists), and privacy (Midjourney was trained on millions of images that the parent company may not have had permission to use).
Further to this, some language models have exhibited worse gender bias. The report notes that most AI firms disproportionately choose to deploy conversational AI systems as female. According to critics, this practice leads to women being the “face” of malfunctions caused by AI defects.
With these and other concerns posed by AI and other potential risks associated with it, we must pay attention to AI principles and prioritize legislative and structural reform over technology solutions, and institutional reforms such as regulatory processes for AI applications.